Researchers at Meta have created a new artificial intelligence tool, Sphere, that can access 134 million web pages and use them as a knowledge base for building AI systems. The first organisation to use the tool, which is being made available on an open-source licence, is Wikipedia, which will deploy it to scan hundreds of thousands of citations on the online encyclopedia to check they support the corresponding claims.
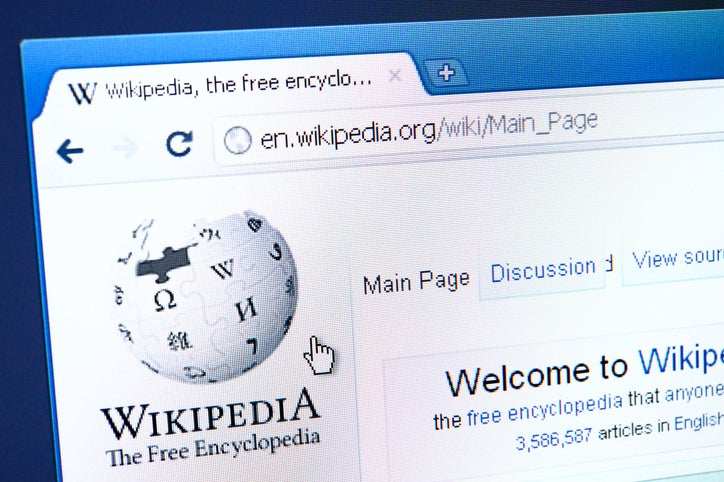
The dataset that can be accessed through Sphere is an order of magnitude larger than any previously released for AI research, Meta claims. For Wikipedia, it will call attention to questionable citations, highlighting those that human editors need to evaluate and change. If the citation proves irrelevant, the model can also suggest other applicable sources of information that do back up the claim in the text.
How will Meta Sphere work with Wikipedia?
Wikipedia relies heavily on citations written in the footnotes of its articles to verify claims made within the text. Its 6.5 million articles are updated regularly by volunteers, and sometimes the citations don’t back up the claims being made.
Using Sphere, Meta says the goal is to provide a platform for Wikipedia editors that can “systematically spot citation issues” and correct the citation or the content of the corresponding article at scale – rather than requiring manual trawls post-by-post.
Related
AI and automation
UK’s ‘collaborative’ approach to AI regulation may prove complex and burdensome
AI and automation
UK government to set out AI regulation plans
AI and automation
IBM acquires Databand to improve machine learning models
AI and automation
Vodafone pens ‘risky’ AI deal with Google Cloud
The tools are built on the back of an existing Meta AI model that integrates information retrieval and verification. It involved training neural networks to learn more nuanced representations of language to pinpoint source material. The latest changes to this involved significantly increasing the size of the pool of data the model can draw from.
This new version of the model, Sphere, references up to 134 million web pages. For Wikipedia, Meta fed it with four million claims from the online encyclopedia, teaching it to zero in on a single source from the vast pool to validate each statement.
The index produced in this process passes potential sources for a Wikipedia article on to an evidence-ranking model that compares the text to the citation and determines whether the citation matches and is a viable option for inclusion in the footnotes.
Content from our partners
How can digital technologies and big data transform the travel experience?
How clinical trials infrastructure is undergoing digital transformation
Webinar – Top 3 Ways to Build Security into DevOps
“Usually, to develop models like this, the input might be just a sentence or two,” a Meta statement said. “We trained our models with complicated statements from Wikipedia, accompanied by full websites that may or may not support the claims. As a result, our models have achieved a leap in performance in terms of detecting the accuracy of citations.”
The implications of Sphere for Meta and Wikimedia Enterprise
All of this work acts to improve Sphere and will in turn potentially allow for new AI systems that can make sense of the real world, according to Meta.
“Open source projects like these, which teach algorithms to understand dense material with an ever-higher degree of sophistication, help AI make sense of the real world,” a Meta blog post said. “While we can’t yet design a computer system that has a human-level comprehension of language, our research creates smarter, more flexible algorithms. This improvement will only become more important as we rely on computers to interpret the surging volume of text citations generated each day.”
Indeed, the company also argues that the breadth of sources used by Sphere means it provides more accurate results than other comparable systems. “Because Sphere can access far more public information than today’s standard models, it could provide useful information that they cannot,” the blog post added.
For the Wikimedia Foundation, the non-profit organisation which oversees Wikipedia, ensuring accuracy is more important than ever before. Last month it launched Wikimedia Enterprise, a commercial product for businesses that require a high level of access to its databases.
Shani Evenstein Sigalov, a lecturer and researcher at Tel Aviv University, and vice-chair of the Wikimedia Foundation’s board of trustees described the new technique as a “powerful example of machine learning tools that can help scale the work of volunteers.”
“Improving these processes will allow us to attract new editors to Wikipedia and provide better, more reliable information to billions of people around the world,” he says.
Read more: Knowledge management is the fastest-growing area of AI spend
Topics in this article: Meta