In an era of accelerated digitalisation, artificial intelligence (AI) and machine learning (ML) have fast become part of the IT infrastructure of many businesses. Consequently, how these technologies are being used to derive meaningful insights from vast quantities of data is maturing rapidly.
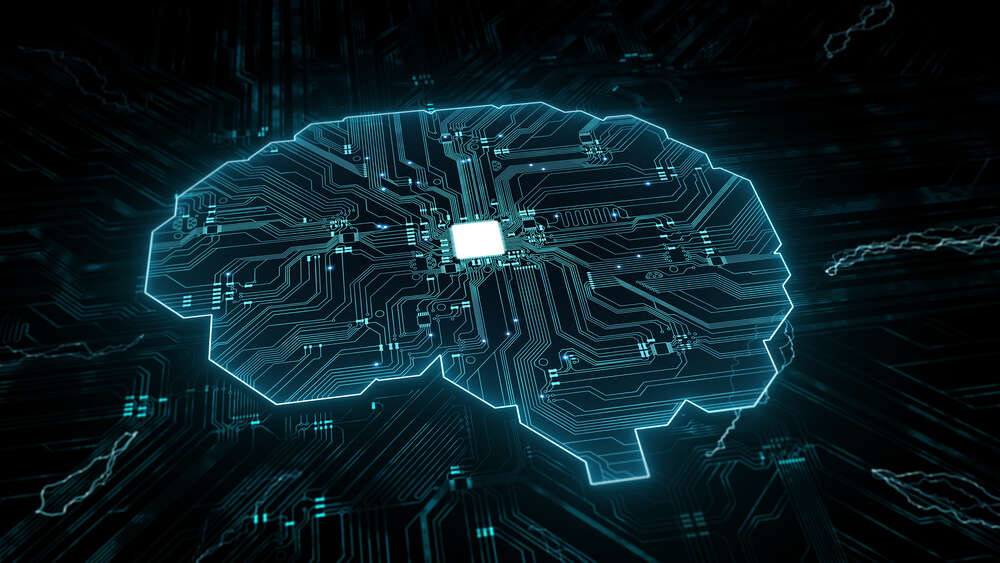
“Early on, when organisations didn’t have access to the computing power and zettabytes of data that they have today, AI was only springing up in pockets,” says Vaidya JR, SVP and global head of data and AI at IT transformation specialist Hexaware Technologies, “The approach then was to see what AI could do for a company, without truly identifying a well-defined problem. Data science solutions were just a shot in the dark.
“Organisations were struggling to put their data to effective use, which led to limited value generated and ineffectual business results,” he adds. “You can crunch any amount of data, and create numerous models; it only adds value if there is a significant impact on the business. But the current attitude has completely changed across industries, without exception.”
From being data-rich but insight-poor, businesses are putting vast amounts of data to work – sensor data, satellite imagery, web traffic, digital apps, video images, customer behaviour metrics and much more. They are in the process of automating and democratising AI and ML, but the attitude now is one of identifying business problems to solve before implementing these technologies. This marks a significant shift: AI and ML strategies are no longer driven by tech, but by strategic business objectives.
Free White Paper
Using AI to Optimize IoT Data in Quality Control for a Global Heavy Vehicle Manufacturer
By Hexaware-TechnologiesEnter your details to receive the free paper:
By downloading this whitepaper, you acknowledge that New Statesman Media Group may share your information with our white paper partners/sponsors who may contact you directly with information on their products and services. Visit our privacy policy for more information about our services, how New Statesman Media Group may use, process and share your personal data, including information on your rights in respect of your personal data and how you can unsubscribe from future marketing communications. Our services are intended for corporate subscribers and you warrant that the email address submitted is your corporate email address.
Thank you. Please check your email to download the white paper.
“Enterprises are looking for use cases to drive business goals and produce results,” observes Vaidya. “AI has to derive efficiency gains at a reduced cost with actionable insights.
“Advanced analytics, AI and ML adoption have skyrocketed across organisations that are seeking to unlock value from data,” he adds. “The race has begun. Everybody is now thinking of democratising AI because they have humongous amounts of data, and it is not humanly possible to crunch all that data manually. So, automation and democratisation are a must.”
Data drives business
In the eyes of the Hexaware executive, this marks a wider corporate trend: in the age of digitalisation, all enterprises are becoming data companies.
Today, automation features across the entire data value chain. The emergence of AI-driven analytics solutions has aided industries in creating greater business value.
“The manufacturing industry, for instance, is fast emerging as completely data-driven,” remarks Vaidya. “We’re talking about digital twins, robots making robots, autonomous cars, and much more. The underlying aspect that is powering the entire possibility is data and AI.
“For example, when you have high rejection rates in your production unit, you need to deploy a parts failure prediction model,” he continues. “You must convert the business problem into a data science problem. What kind of data is required to iterate on the model and improve its accuracy and reliability? How should it be deployed in the real world? These are the areas where we step in and help our customers across industries figure things out.”
Even in professional services, Vaidya says, the linear models are long gone: “It is no longer about a consulting firm approaching the client, building a team, and delivering people-based solutions. Instead, they are turning towards self-service platforms. We’re looking at data platforms customers can subscribe to, that provide automation to the extent where customers only need to identify their problem and the necessary parameters, after which these self-service platforms process their requirements. This is applicable to numerous industries, like auditing, advisory, accounting, healthcare, insurance and more.”
“Data-driven customer experience is another area many of our customers are prioritising, using omni-channels for hyper-personalisation, and customising their journeys in real time,” he adds. “AI recommendation engines for personalisation are extensively used across industries to recommend a customer’s next purchase or next show to watch based on their past purchases or watch history.”
From the acquisition of data to the gleaning of insights, be it through embedding models or operationalising, automation is increasingly prevalent across the data value chain. Enterprises are increasingly turning to automated machine learning (AutoML). This process automates time-consuming, iterative tasks of ML model development, and also serves towards democratising machine learning.
“In fact, AutoML will eventually reach a stage where if a business use case is given, it will identify options regarding how to convert that case into a typical data science problem, all the way up to operationalising AI,” predicts Vaidya.
Building ML models is only a part of the process. Operationalising them is where MLOps – a set of practices for reliably and efficiently deploying and maintaining ML models in production, transitioning the algorithms to production systems – comes into the picture.
Artificial intelligence partnerships power
With AI strategy driven by the C-suite, rather than the IT department, third-party partners like Hexaware have a crucial role to play in helping customers deliver digital initiatives and build connected customer-centric digital enterprises.
“From our experience, digital enterprises are characterised by certain factors – hyper-personalisation, connected enterprises, customer-centric and advanced production methods,” says Vaidya. “We understand the transformations being witnessed in the market, compare their peers in the industry and then engage with our clients to co-create value. We also help organisations understand how transforming the digital helps transform the physical. Edge computing, PaaS, servitisation, IoT and IIoT – in the B2B segment – are only a few of the technologies that help make that connection.
“Where we step in is at the conversion stage. We help build digital enterprises, by converging technologies and deriving the best from them. This includes creating a data architecture roadmap, an AI Center of Excellence (CoE) roadmap, change management and guiding enterprises on how their consultants can be reskilled. We create and build a minimum viable product quickly that they can test through the entire value chain.”
Vaidya acknowledges that change is happening at an ever-increasing pace, but cautions that businesses must be careful not to rush. A foundation must first be set, instead of jumping on the AI bandwagon for the sake of it. “We start from scratch, preparing organisations for business change,” he explains. “Right from identifying the business challenge and use cases, to converting them into data science problems, to eventually building an agile data engineering organisation, which is a fundamental piece, since your model is only as good as the data it gets.”
There are numerous adoption challenges that enterprises might face, right from excessive costs borne owing to inaccurate models, lack of skilled SMEs or even identifying the right data sets.
Ultimately, it boils down to understanding the business, defining the business challenge at hand, and converting it into a data science problem. This requires a team encompassing the right blend of skills, roles and responsibilities, coming together to create an ecosystem that generates value repeatedly and reliably.
Topics in this article: AI, Analytics, Hexaware Technologies, Machine Learning, sponsored