The UK’s National Cyber Security Centre (NCSC) has published a set of security principles for developers and companies implementing machine learning models. An ML specialist who spoke to Tech Monitor said the principles represent a positive direction of travel but are “vague” when it comes to details.
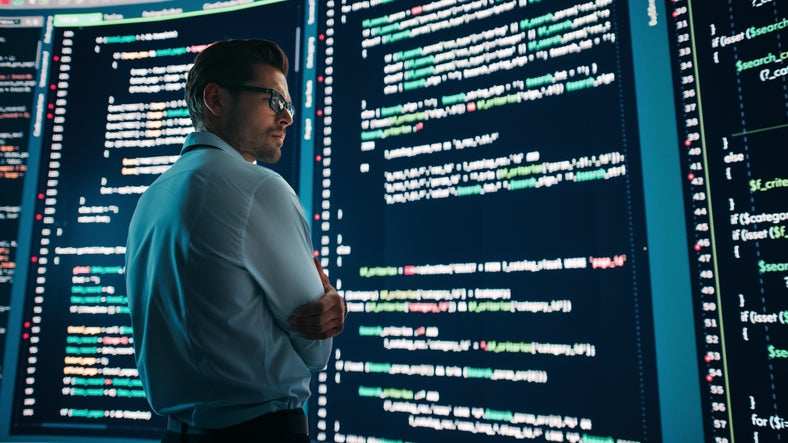
The NCSC has developed its security principles as the role of machine learning and artificial intelligence is growing in industry and wider society, from the AI assistant in smartphones to the use of machine learning in healthcare. The most recent IBM Global AI Adoption Index found that 35% of companies reported using AI in their business, and an additional 42% reported they are exploring AI.
The NCSC says that as the use of machine learning grows it is important for users to know it is being deployed securely and not putting personal safety or data at risk. “It turns out this is really hard,” the agency said in a blog post. “It was these challenges, many of which don’t have simple solutions, that motivated us to develop actionable guidance in the form of our principles.”
Doing so involved looking at techniques and defences against potential security flaws, but also taking a more pragmatic approach and finding actionable ways of protecting machine learning systems from exploitation in a real-world environment.
Companies Intelligence
View All
Reports
View All
Data Insights
View All
The nature of machine learning models, which sees them evolve through automatically analysing data, means they are difficult to secure. “Since a model’s internal logic relies on data, its behaviour can be difficult to interpret, and it’s often challenging (or even impossible) to fully understand why it’s doing what it’s doing,” the NCSC blog says.
This means many machine learning components are being deployed in networks and systems without the same high level of security found in non-automated tools, leaving large parts of the system inaccessible to cybersecurity professionals. This in turn is causing some vulnerabilities to be missed and exposes the system to attack and is also introducing more vulnerabilities that are inherent in machine learning and are present throughout all stages of the machine learning lifecycle.
Lack of transparency in machine learning models
The group of attacks that are designed to exploit these inherent issues in machine learning are known as “adversarial machine learning” (AML) and understanding them requires knowledge of multiple disciplines including data science, cybersecurity, and software development.
The NCSC produced a set of security principles for systems containing ML components with the goal of bringing awareness of AML attacks and defences to anyone involved in the development, deployment or decommissioning of a system containing ML. The logic used by ML models and data used to train the models can often be opaque, leaving security experts in the dark when it comes to inspecting them for security flaws.
Content from our partners
How collaborative automation can accelerate digital transformation
Are you ready to respond to the latest cyber threats in education?
A blueprint for solving merger and acquisition HR challenges
The principles suggest designing for security when drafting system requirements, securing the supply chain and making sure data comes from a trusted source and securing infrastructure by applying trust controls to anything and anyone that enters the development environment.
Assets need to be tracked through the creation of documentation covering the creation, operation and lifecycle management of models and datasets, and ensuring the model architecture is designed for security.
“ML doesn't necessarily pose any more danger than any other piece of logic in a software system but there are a few nuances to ML models that should be appreciated,” says Nigel Cannings, the founder of compliance solution company Intelligent Voice.
“An ML system is built on data, the finished system represents valuable intellectual property and in some cases, the data used to train it is also something that is often needed to be protected.”
This mirrors the concerns raised by the NCSC which said that without open information on the data used to train machine learning algorithms or the methods it uses to make its findings, it is difficult to spot vulnerabilities that could expose the system.
However, Cannings warns that while the NCSC principles are a positive move, the lack of detail makes them less useful as a tool for communicating potential risks. “The principles in the NCSC are vague, and provide general guidelines with much borrowed from conventional software cybersecurity," he says. "They are not wrong and point to the importance of education of developers and data scientists but more detail could have been provided to communicate the risks."
NCSC ML security principles 'a direction of travel'
Developers and admins are likely to take steps to protect their models if they are aware of the risks they can expose, explains Canning, adding that “in the same way software engineering has evolved to be increasingly more security conscious, ML and MLOps will benefit from this practice too.”
The NCSC principles are more a “direction of travel” than a set of guidelines or blueprint to follow, he says, and the exact measures taken will vary by model and change with research.
Todd R Weiss, an analyst for Futurum Research adds: “It is wise to consider all aspects of security when it comes to AI and ML, even while both technologies can also help companies address and solve technology challenges. Like so many things in life, AI and ML are also double-edged swords that can bring huge benefits as well as harm. Those concerns must be balanced with their benefits as part of an overall IT infrastructure and business strategy.”
Despite these inherent risks, Weiss said AI and ML are “far more beneficial and useful as technologies in our world”. He argues: “Without AI and ML, incredibly powerful digital twins would not be possible, medical breakthroughs would not be happening and fledgling metaverse communities would not be possible. There are many other examples of the benefits of AI and ML, and there will always be bad actors searching for ways to cause havoc in all forms of technology."
Weiss praised the NCSC for its ML security principles as they will “encourage awareness, acceptance, and critical thinking about these ongoing concerns and can actively help businesses truly take these matters to heart when using and exploring AI and ML”.
Read more: Meta has questions to answer about its responsible AI plans
Topics in this article: AI, Cybersecurity